機械学習は血液透析中の透析中低血圧を予測できる
臨床医が測定する標準的な各種臨床変数を解析し、機械学習法による透析時低血圧の予測能を判定
英ポーツマス大学のShamsul K. Masum氏らは、臨床医が測定する標準的な各種臨床変数を解析することで、機械学習法によるIDHの予測能を検討した。2000~2020年に10施設で実施された7万3,323回の血液透析(患者数3,944人、IDH 3万6,662件)のデータセットを対象に解析を実施した。
解析の結果、収縮期血圧(SBP)と拡張期血圧(DBP)が重要な予測因子であることが分かった。IDH患者は、IDHが認められなかった患者よりも透析前のSBPが低く、透析中のSBP低下率が大きかった。同様に、IDH患者は透析前のDBPが低く、透析中のDBP低下率が大きかった。透析前のSBPが低く、透析中のSBP低下幅が大きく、体重減少幅が大きいほど、IDHの発生率が高くなった。特異度が最も高く(73.9%)、全般的予測精度が高かった(75.5%)のは、ランダムフォレストを用いた機械学習モデルであった。しかしながら、IDH予測感度が最も高かった(78.5%)のは、双方向長短期記憶(Bi-LSTM)を用いたモデルであった。別の検証データセットにおけるBi-LSTMモデルの精度は74.09%、感度は74.81%、特異度は73.3%、ROC曲線下面積(AUROC)は0.816であった。透析前のデータのみを用いた場合は予測能が低下し、精度が68.60%、特異度が69.81%、感度が67.40%、AUROCが0.757であった。
著者らは、「機械学習アルゴリズムを用いた予測モデルは、IDHのリスクがある患者を事前に特定するための非常に有望なツールとなるだろう」と述べている。
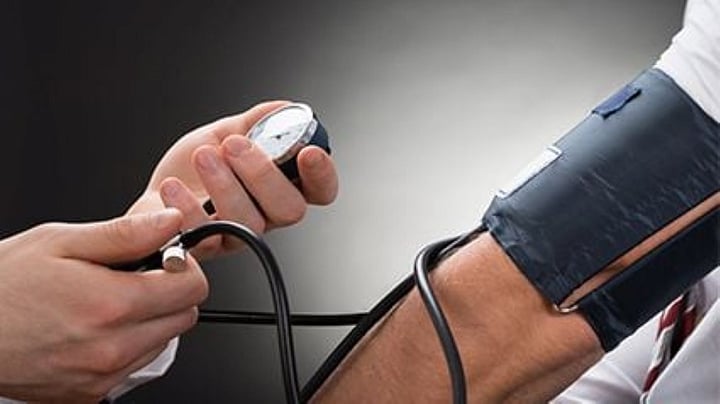
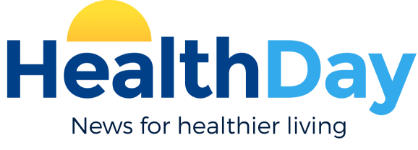
Machine Learning Can Predict Intradialytic Hypotension During Hemodialysis
Various standard clinical variables measured by clinicians analyzed to determine scope of machine learning techniques in predicting IDH
WEDNESDAY, Nov. 6, 2024 (HealthDay News) -- Clinical data and machine learning can help to predict intradialytic hypotension (IDH) for patients undergoing hemodialysis, according to a study published online Sept. 14 in the Journal of Kidney Care.
Shamsul K. Masum, Ph.D., from the University of Portsmouth in the United Kingdom, and colleagues investigated the scope of machine learning techniques in predicting IDH by analyzing various standard clinical variables measured by clinicians. The dataset included 73,323 hemodialysis sessions (3,944 patients) with 36,662 IDH events seen at 10 centers during 2000 to 2020.
The researchers found that systolic blood pressure (SBP) and diastolic BP (DBP) were key predictor variables. Predialysis, patients with IDH had lower SBP, as well as a greater percentage drop in SBP during dialysis. Similarly, people with IDH had lower predialysis DBP and a greater percentage drop in their DBP. IDH probability increased with lower pre-SBP, greater delta-systolic, and increased weight loss. Highest specificity (73.9 percent) and highest overall predictive accuracy (75.5 percent) were seen with a machine learning model with Random Forest. However, a model with Bidirectional Long Short-Term Memory (Bi-LSTM) achieved the highest sensitivity (78.5 percent) in predicting IDH. In a separate validation dataset, the Bi-LSTM model achieved accuracy of 74.09 percent, sensitivity of 74.81 percent, specificity of 73.3 percent, and a receiver operating characteristic-area under the curve (ROC-AUC) of 0.816. Based only on predialysis data, the prediction performance dropped to accuracy of 68.60 percent, specificity of 69.81 percent, sensitivity of 67.40 percent, and an ROC-AUC of 0.757.
"A prediction model using machine learning algorithms offers great promise as a tool in identifying patients at risk of IDH in advance," the authors write.