疑わしい皮膚病変の早期発見に有効な新たなリスク因子群を特定
これらの因子を用いたAIモデルはが感度80.46±2.50%、特異度62.09±1.90%を達成、他の評価法を上回る
英エセックス大学のShafiqul Islam氏らは、病変のサイズや形状、患者の年齢や性別など23項目の属性から成るメタデータを用いた、疑わしい皮膚病変を検出する人工知能(AI)モデルを検討した。今回新たに特定された最も重要な皮膚がんのリスク因子群「C4Cリスク因子」について、その精度を、メラノーマの早期発見のために広く使用されている7-point checklist(7PCL)およびメラノーマの生涯リスクを予測するためのWilliamsリスク因子と比較した。
患者のメタデータを組み込んで設計されたAIモデルにより、7つの新たな皮膚がんリスク因子(ピンク色の病変、病変のサイズ・色・炎症・形状・経過年数、地毛の色)が特定された。このAIモデルで、複数の専門クリニックから収集した5万3,601個の皮膚病変に関するメタデータを評価したところ、疑わしい皮膚病変の検出精度は、感度80.46±2.50%、特異度62.09±1.90%を達成し、7PCLの感度68.09±2.10%、特異度61.07±0.90%、およびWilliamsリスク因子の感度66.32±1.90%、特異度61.71±0.6%を上回った。最良の精度は、C4Cリスク因子を7PCLおよびWilliamsリスク因子と組み合わせることによって達成され、感度85.24±2.20%、特異度61.12±0.90%であった。
著者らは、「今後の研究では、今回新たに特定されたリスク因子群およびその加重リスクスコアであるC4Cリスクスコアを、ディープラーニングモデルを用いて病変画像と融合させることで発展させていく予定だ。これにより費用対効果の高い方法で皮膚がん検出の精度をさらに向上させることができると考えている」と述べている。
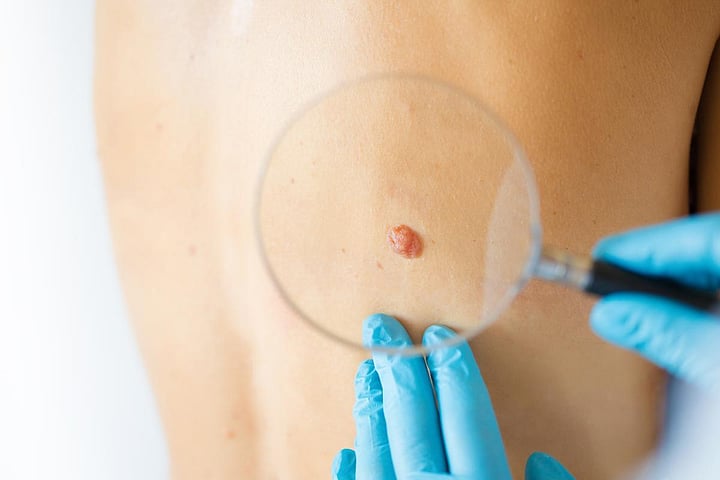
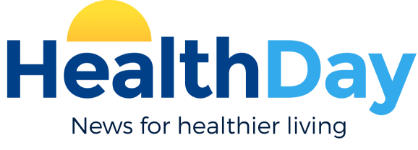
New Set of Risk Factors Can Boost Detection of Suspicious Skin Lesions
C4C risk score achieved sensitivity of 80.46 ± 2.50 percent and specificity of 62.09 ± 1.90 percent, outperforming other models
MONDAY, Nov. 4, 2024 (HealthDay News) -- A new set of skin cancer risk factors can improve detection of suspicious skin lesions, according to a study published online Sept. 6 in Scientific Reports.
Shafiqul Islam, Ph.D., from the University of Essex in Colchester, England, and colleagues examined artificial intelligence (AI) models that use patient metadata consisting of 23 attributes for suspicious skin lesion detection. Performance of a new set of most important risk factors, known as C4C risk factors, was compared to that of the 7-point checklist (7PCL) and the Williams risk factors that predict lifetime melanoma risk.
The proposed AI framework identified seven new skin cancer risk factors: lesion pink, lesion size, lesion color, lesion inflamed, lesion shape, lesion age, and natural hair color. The researchers found that when evaluating metadata from 53,601 skin lesions collected from different skin cancer diagnostic clinics, this proposed framework achieved sensitivity of 80.46 ± 2.50 percent and specificity of 62.09 ± 1.90 percent for detecting suspicious skin lesions compared with 68.09 ± 2.10 percent sensitivity and 61.07 ± 0.90 percent specificity for 7PCL and 66.32 ± 1.90 percent sensitivity and 61.71 ± 0.6 percent specificity for the Williams risk factors. The best performance was achieved by combining the C4C risk factors with 7PCL and Williams risk factors, with 85.24 ± 2.20 percent sensitivity and 61.12 ± 0.90 percent specificity.
"In our current and future research, we are extending our investigation through the fusion of the newly identified skin risk factors and weighted risk score together with lesion images using deep learning models, which we believe will further boost the performance of skin cancer detection in a cost-effective manner," the authors write.